The Claim:
RFK Jr. is getting a lot of attention for his claims that SARS-CoV-2 was engineered to attack some while being less severe for others. His history of claiming that vaccines are prone to causing autism, particularly in some sub-segments of the population, is being recirculated by people who agree and feel like now is the moment.
The Facts:
RFK Jr.’s claims about autism are based on a re-analysis by Brian Hooker (chemical engineer) of a previous autism study. One of its co-authors of the original study expressed concerns about the original findings, claiming that important data was left out. So, Hooker got the data he claimed was missing, added it to the study, and did his own calculations.
The CDC defended its original study and said there was no cover-up.
Experts criticized Hooker’s re-analysis, saying it was flawed and didn’t consider other variables that could influence the results. They questioned how he used the CDC data set and argued that the study design was inappropriate for his analysis.
Brian Hooker used a cohort design to analyze a dataset originally intended for case-control analysis. Subsequently, he performed multiple subset analyses, which can besusceptible to false positives.
When evidence is excessively segmented or divided into smaller subsets for analysis, there is a higher likelihood of observing apparent correlations that may not nactually be meaningful or valid. This phenomenon is often called the “multiple comparisons problem” or “data dredging.”
Let’s illustrate this with an example:
Imagine a researcher is studying the relationship between eating ice cream and the incidence of sunburns in a particular city over the summer months. They collect dataon ice cream sales and sunburn cases for each day of the summer and decide to analyze the data in several ways:
- They compare ice cream sales on sunny days vs. cloudy days to see if more ice cream is sold on sunny days. They find a correlation: more ice cream is sold on sunny days.
- They analyze sunburn cases on sunny days vs. cloudy days to see if more sunburns occur on sunny days. They find a correlation: more sunburns occur on sunny days.
- They break the data into different age groups and compare ice cream sales and sunburn cases for each group. They find correlations within certain age groups.
- They split the data into different time intervals (morning, afternoon, and evening) and compared ice cream sales and sunburn cases for each interval.
They find correlations within some time periods.
Now, the researcher has several apparent correlations:
- Sunny days are correlated with higher ice cream sales.
- Sunny days are correlated with more sunburn cases.
- Certain age groups show correlations between ice cream sales and sunburn cases.
- Specific time intervals show correlations between ice cream sales and sunburn cases.
But here’s the catch: just because these correlations appear in the data doesn’t necessarily mean a true cause-and-effect relationship exists between eating ice cream and getting sunburned. The correlations might be coincidental and not based on any real underlying relationship.
In scientific research, it is crucial to be cautious about interpreting correlations.
And about taking medical advice from environmental lawyers.
Disclaimer: Science is always evolving and our understanding of these topics may have evolved too since this was originally posted. Be sure to check out our most recent posts and browse the latest Just the Facts Topics for the latest.
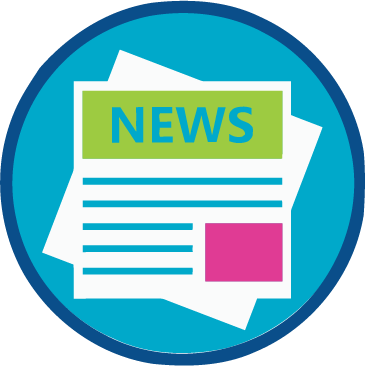
Just the Facts Newsletter:
Correcting this week's disinformation
Sign up to get a weekly look at the latest vaccination facts as we debunk the latest false vaccination claims making the rounds on the internet.